Single Cell Biology
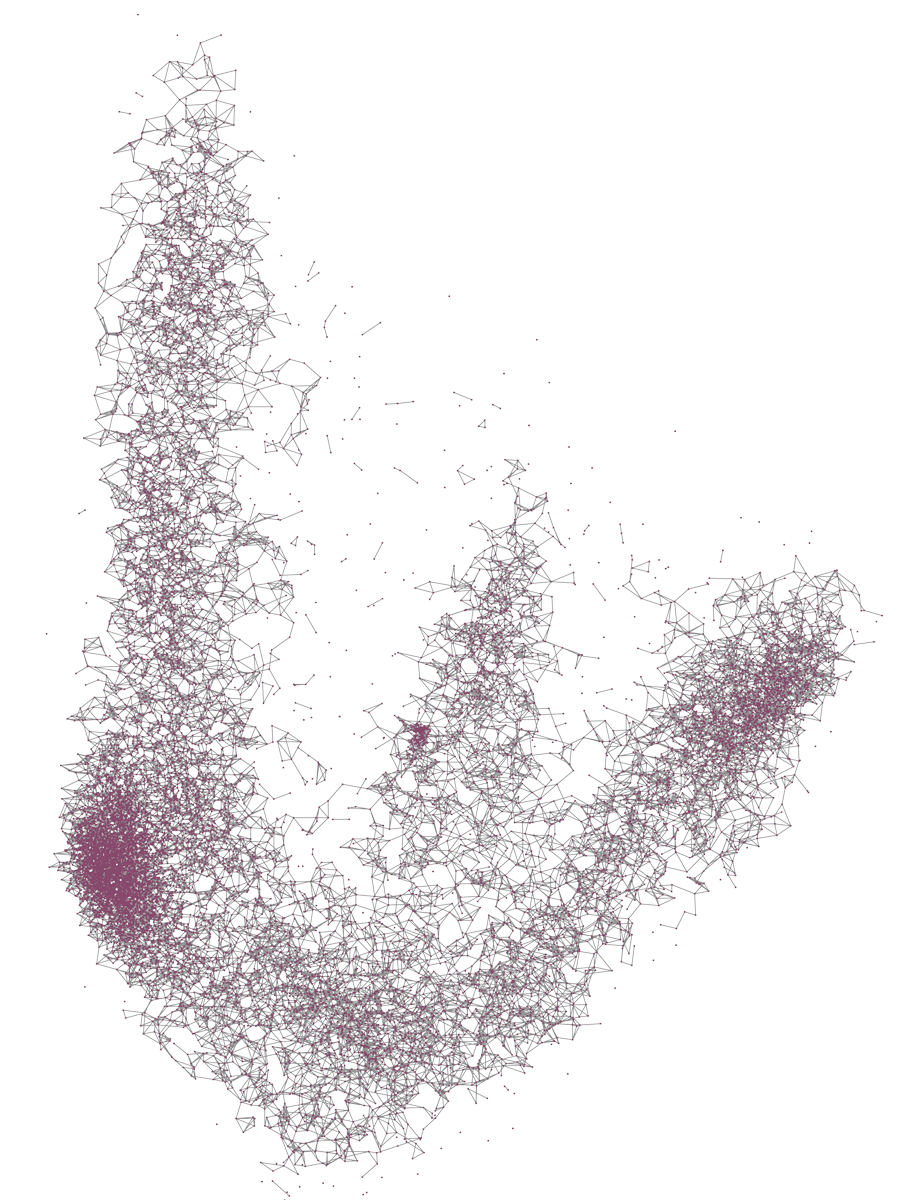
Cell Biology At Scale
The human body emerges through a remarkable process of cellular self-organization where billions of cells move, divide, change shape, communicate, and differentiate to construct complex three-dimensional tissues like the brain.
Biology has undergone a measurement revolution in which we can now profile the global state of thousands of individual cells—measuring 20,000 genes at a time in thousands of single cells per experiment.
We are developing machine learning methods that build predictive models from these massive-scale single cell data sets. We then test these models by devising strategies to explicitly control and rewire tissue self-organization.
PopAlign
We’ve introduced a mathematical modeling platform, PopAlign, that categorizes and analyzes gene expression changes that occur in complex mixtures of single cells as they respond to signals, drugs, or disease states.
PopAlign constructs a probabilistic model of each cell population across a series of samples. PopAlign then automatically identifies and models subpopulations, aligns these cellular subpopulations across experimental conditions (i.e. signaling, disease) and quantifies changes in cell abundance and gene expression for all subpopulations of cells.
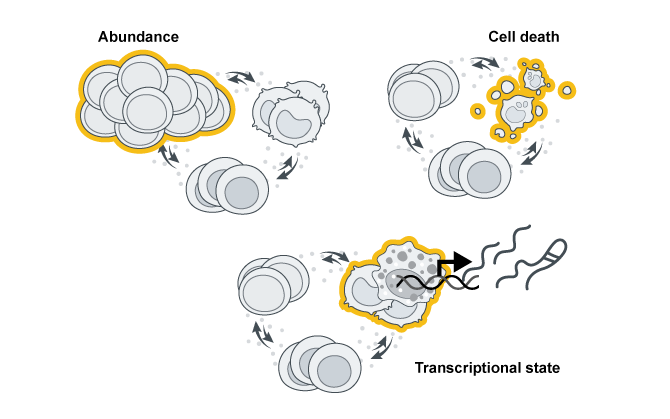
Interacting single cell populations respond to perturbations.
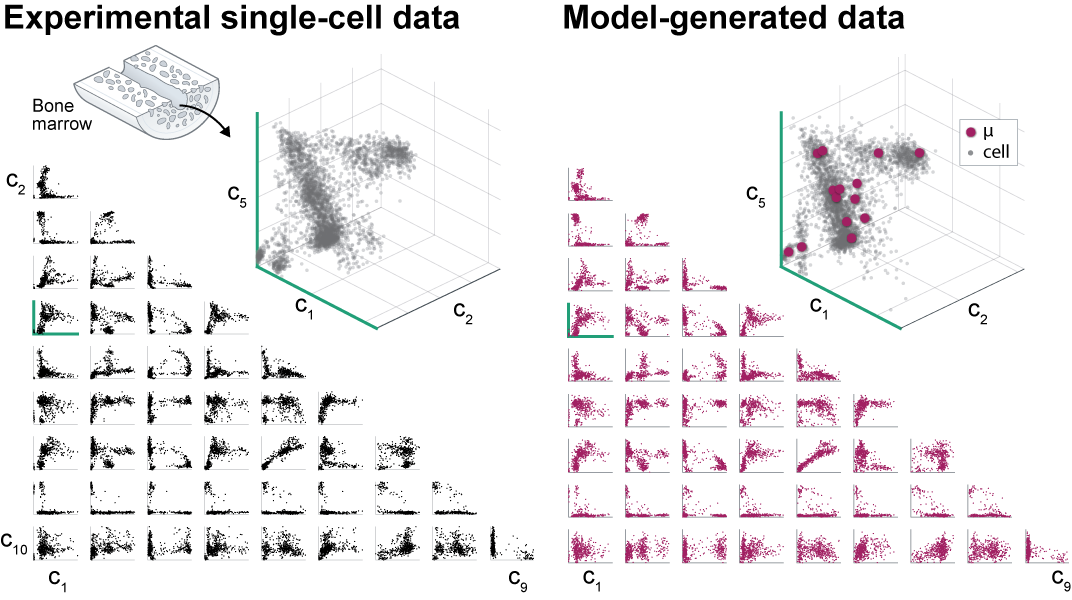
Pop-Align data aligns with single-cell data.
PopAlign scales to comparisons involving hundreds of samples, enabling large-scale studies of natural and engineered cell populations as they respond to drugs, signals or physiological change, reducing the memory footprint of a typical 10,000 cell sample by up to 100x.
Algorithmic Tissue Engineering
Employing the PopAlign mathematical platform and our active matter models, we are developing an integrated experimental and computational platform for constructing and testing computational models of tissue organization.
We are developing a new framework that builds a candidate model of a network, generates an optimal set of perturbation experiments that can be applied to test and refine this model, and updates the model structure through iterative rounds of data collection, modeling, and perturbation. We’re working towards an efficient distributed computing architecture that can simulate millions of interacting cells.
This computational, engineering driven approach will identify protein regulatory networks that control the behavior of single cells. Predictive models could reveal fundamental principles for tissue self-organization and initiate a new area of algorithmic tissue engineering where repair and engineering strategies are optimized through simulation.
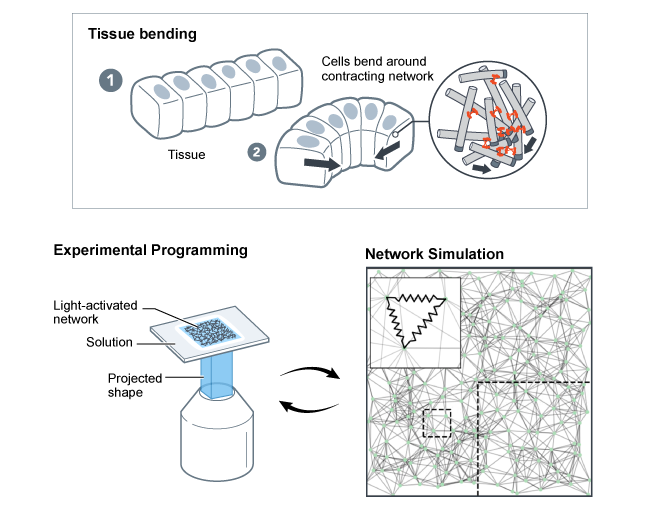
Simulated networks give insight into the physical mechanisms involved in tissue bending.